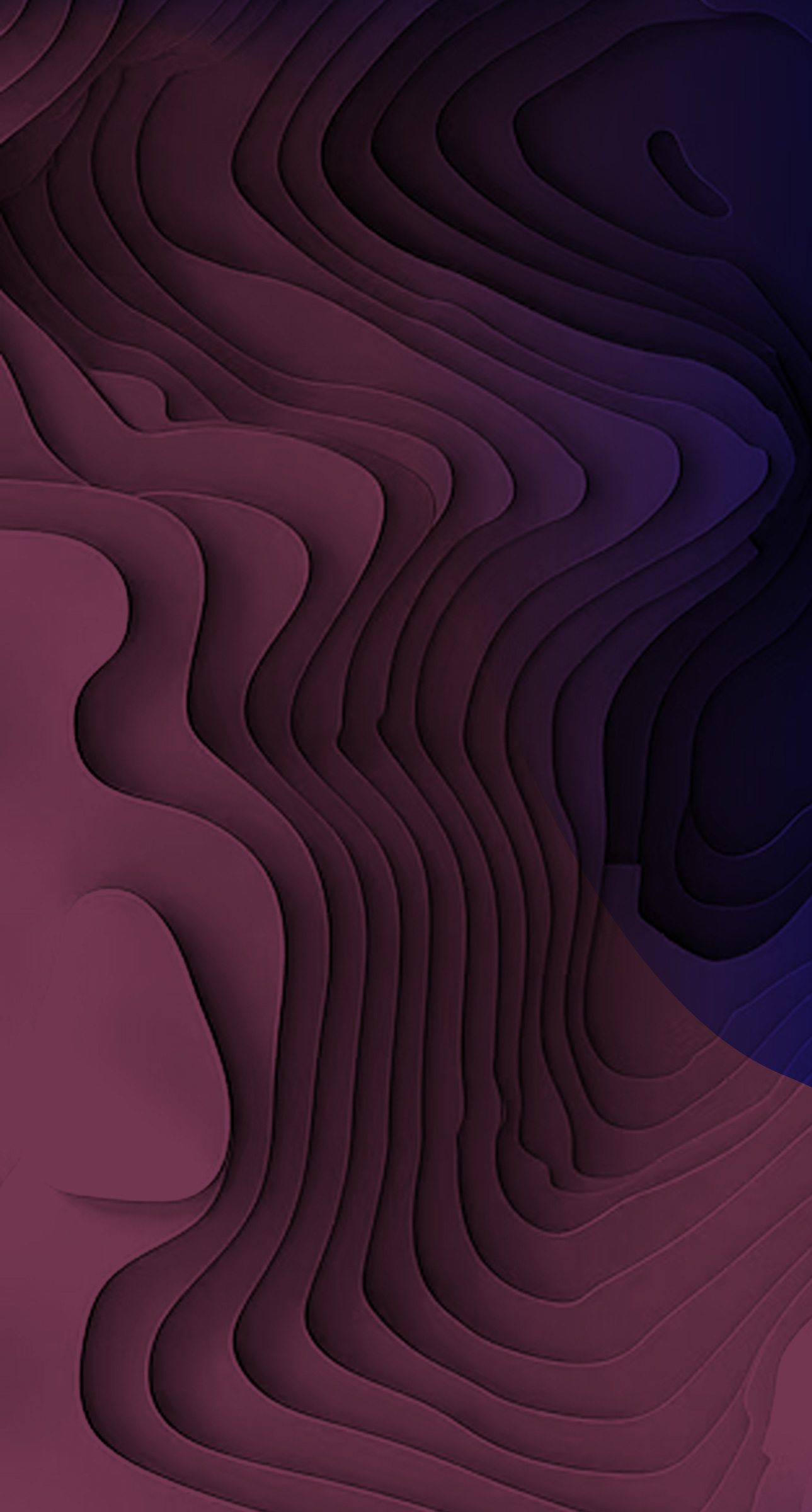
The abstraction from this biologic example is that utilizing more data to attack a problem leads to a solution set of significantly better local and global maxima. We believe this will vastly improve the human condition. If we view the data underlying a problem-answer set as creating a landscape of outputs with varying levels of efficacy or peaks then the larger (and richer) the geography to search (ie the bigger and better the data sets) the likelihood of finding a (much) better peak goes up – as long as we have the analytics and compute power to search effectively. Indeed data collection and computation go hand-in-hand to solve problems and create value.
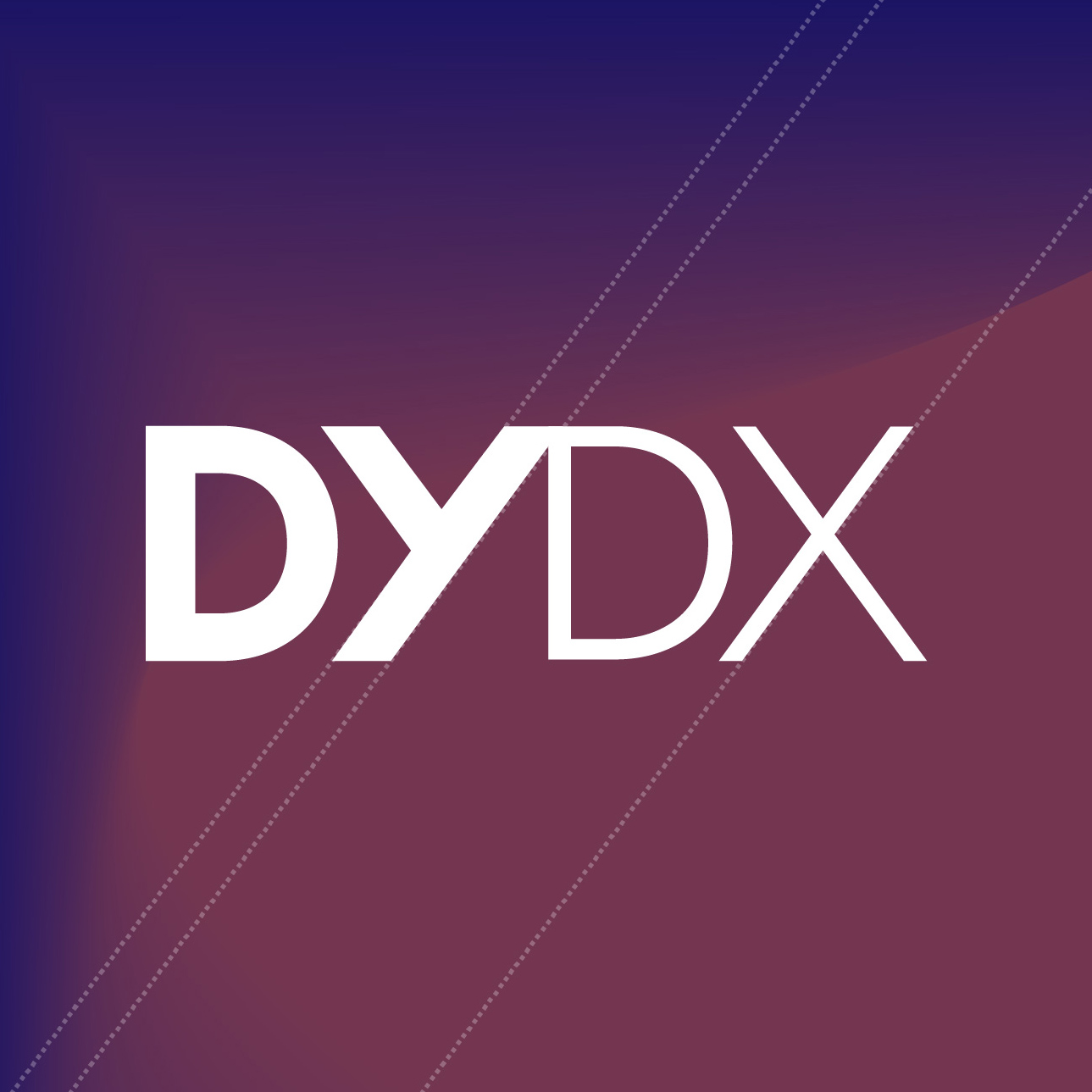
Fortunately both data collection and compute power have greatly improved. As IOT devices, sensors and imaging have all improved (both in cost efficiency and capability) the amount of data has concomitantly increased exponentially. Similarly at the front of this has been Moore’s law and an exponential increase in the power of processors increasing the number of computations that can be performed in a given amount of time to more rapidly exhaust a search space. A starting point for leveraging the value of these data streams that were coming online was to exploit easily structured data. Thus the rise of Oracle, SAP and others that built the software infrastructure for relational databases to house structured data and find correlations to create actionable outcomes. These were the underpinnings of the first wave of data-driven enterprise software.
However most data is difficult to use because it is unstructured data, such as images, text and a lot of scientific data. This data is valuable because it is very rich and hence increases the solution search space exponentially. However to use this data it must first be structured in some way. Post the financial crisis in 2008 advanced data science began to make large inroads in dealing with these data sets. We demarcate the year 2014 as an inflection point for data science and machine learning tools. Subsequently in 2022 ChatGPT, and the age of AI, was a new bit flip for data science where huge unstructured data sets could now be organized, analyzed and used to build models that could allow machines to do new, previously untaught things. We are rapidly moving toward a world where computation will find peaks that we don’t understand.
[1] Schena, M., Shalon, D., Davis, R. W. & Brown, P. O. Quantitative monitoring of gene expression patterns with a complementary DNA microarray. Science 270, 467–470 (1995)